Most companies that contact us (Reflective Data) for data services already have specific use cases for their data infrastructure (mainly data pipelines and a data warehouse). Nevertheless, we occasionally get approached by businesses that kind of know they’d need a data warehouse but aren’t entirely sure how to benefit from having one.
Possible use cases are virtually limitless and depend on what kind of business you run. In this article, I’m providing some of the more common ways together with examples of how to benefit from having a data warehouse.
In no specific order, here we go.
Better attribution leads to more optimized marketing and ad spend
It’s no secret that some analytics tools and most ad platforms tend to default to attribution models that work best for them. I mean, they use models that show as if they’re bringing you the most traffic and conversions. Some tools let you modify the model or attribution windows to some extent but generally, it’s quite limited.
Let’s say someone scrolled past your ad on Facebook without clicking on it. Later that day they receive an email from you with a nice offer – they end up visiting your suite and making a purchase. Guess what Facebook will tell where this purchase came from? You guessed it, they will attribute it to seeing the ad on Facebook.
Now imagine having all this attribution data available in your data warehouse in a raw format. This will give you full flexibility over which attribution model and window to use. No longer “apples to oranges” comparisons when it comes to your traffic sources.
Combine this with data from other sources like your CRM or backend and you’ll have the ultimate attribution machine in your hands. Use attribution models like Markov Chain or even AI/ML-based models and use metrics like LTV or churn for long-term analysis.
Proper attribution with an “apples to apples” comparison is the key to optimizing your marketing and ad spend.
Perhaps the best way to start collecting this kind of data in your data warehouse would be Parallel Tracking.
More accurate audiences lead to better targeting and savings in ad spend
What if I told you there was a way to evaluate visitors or groups of visitors based on their likelihood of becoming a customer or even a high-value long-term loyal customer? All before they even land on your website or make their first purchase. Imagine building your advertising audiences based on those predictions while constantly improving the model. Well, the big players have been doing this for years but today the barriers have lowered and most businesses could leverage this kind of technology. Trust me, it’s easier than you might think.
There are three main components to getting started with AI/ML-based audience future value predictions.
- Set up a data warehouse – build yourself or hire Reflective Data to consult and help with the technical setup
- Configure data pipelines (the systems that transfer data from various sources into your data warehouse) – We’ve got you covered if you need help
- Leverage a system similar to BigQuery ML for the predictive models – Yes, we can help with the ML part, too
Instead of spending your precious marketing budget on people that’ll never buy from you, focus on those that have the highest potential of becoming high LTV customers.
$$$
Data-driven product recommendations lead to higher AOV
Product recommendations, if done right, can provide enormous value for both the customers and merchants. Now that we have tools like Recommendations AI from Google, getting started with your own recommendations engine has never been easier. Or cheaper.
All you need is data, preferably in a data warehouse like BigQuery. For this use case, I’d recommend using Parallel Tracking to get your data into your data warehouse.
Case study: How IKEA managed to increase their Click Through Rates by 30% and AOV by 2% by leveraging the Recommendations AI. LINK
Data-driven marketing automation drives more sales
Still sending generic emails to all customers or manually building audiences? Welcome to the modern days where we can leverage the rich customer data available in our data warehouses. Use it to automate many critical parts of our marketing. Including emails.
A few scenarios to consider.
- Predictive model described above detects people with the highest future customer lifetime value (LTV). Nudge those people a bit by sending them an offer that’s a bit more generous than what you’d send to everyone. Believe me, it’s worth it.
- Include personal product recommendations in your marketing emails.
To get high-quality data into your data warehouse, you need a data pipeline that connects with your existing tools.
Data-driven personalization drives more sales and higher AOV
There are many ways how you can introduce personalization to your customers but one aspect is true for all of them – they all need good quality data as input.
Below are a few common personalization solutions our clients have implemented.
- Customize the front page of your e-commerce (or any other) website based on each customer’s previous browsing and shopping behavior. This can include banners, offers, discounts, product recommendations and more.
- Customize the website experience based on the type of customer that has landed on your site. Depends on your business type and target audience but generally individuals, people representing small businesses and those shopping for a large enterprise all expect a somewhat different experience.
- Personalize overall messaging based on visitors’ behavior. Let’s say you’re an insurance company and someone landed on your blog article comparing different car insurance options, then they move on and read a few more related articles. Now, when they land on your main website, it’d be good to welcome them with relevant car insurance options as opposed to generic content.
To get high-quality data into your data warehouse, you need a data pipeline that connects with your existing tools.
Analyzing user behavior can lead to savings in customer support costs
Maintaining a proper support team can be costly. Many companies that we’ve worked with could’ve (and eventually have) saved anywhere from 5% to 60% in support-related costs by having relevant data available in their data warehouse, analyzing it and, of course, making the changes based on insights found.
Data points to collect here include.
- Live chat messages
- Call transcripts
- Site search behavior
- Help center behavior
- Social media, Reddit and other platforms
To collect this kind of data into your data warehouse, you need a data pipeline that connects with the tools necessary.
Potential ways to execute those insights include.
- A/B testing
- Chat bot
- Re-arranging help center
- Train support personnel
- Improve your product UI/UX
- Better onboarding guides
Running an experimentation program on top of a data warehouse will save $ on tool vendor costs
Most conventional experimentation (A/B testing) tools like Optimizely, Google Optimize and others operate in silos and keep their own copy of all the data they need to operate. In reality, though, this dataset is very similar to many other tools that you may already use, including Google Analytics. Having another tool to operate in a silo isn’t (cost) effective and can often lead to data discrepancies.
Modern experimentation tools like Eppo, Growthbook and others don’t create another siloed copy of your data. Instead, they will sit on top of your data warehouse and use data you already have – nicely integrated with various sources. Not only is this much more cost-effective but it also helps you avoid creating yet another source for data discrepancies. Not to mention that you don’t have to define all the goals beforehand as you can simply use SQL to define those after the fact. Or while the test is already running.
A simple data pipeline and an open-source tool like Growthbook is all you need to get started. Save tons compared to tools like Optimizely and get a more robust solution that enables you to test across all parts of your tech stack. Front end, back end, APIs, databases and more.
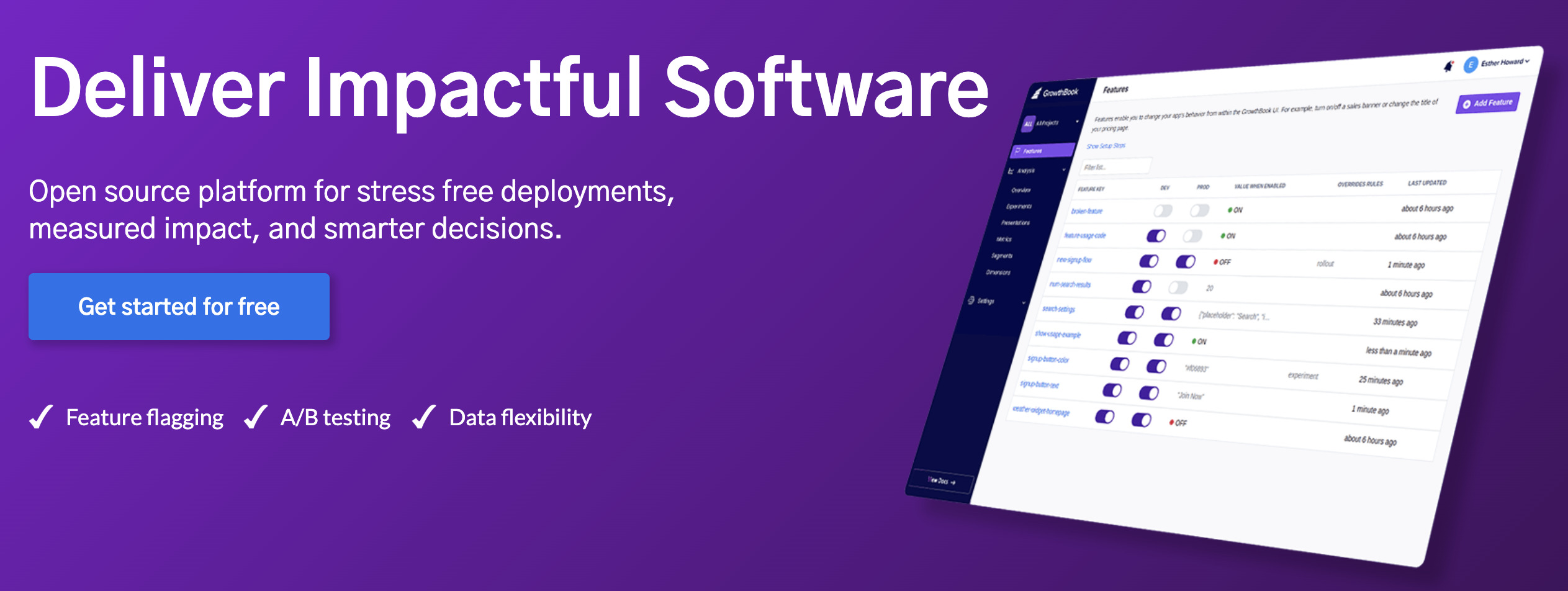
Using a data warehouse in favor of a conventional analytics tool will save $ on tool vendor costs
There are free analytics tools out there but they all come with quite serious limitations which leads most bigger companies to look for a paid solution. What most tools really do is provide you with a tracker, data processing endpoint and a few shiny reports – oh, and ask a fortune for doing so.
In reality, all you really need is a tool like Parallel Tracking that connects with your existing Google Analytics (both UA and GA4) trackers while duplicating hits to another processing endpoint and then sending them straight into your data warehouse. Free from data collection, cardinality or other limitations which are often seen even in the paid analytics platforms. From there, connect your BI tool and build all the reports you might ever need. All while saving like 10x compared to something like GA360.
As a bonus, you’ll likely have data from other sources in your data warehouse, too. This unlocks the potential to do even more advanced analysis that’s not possible with any one analytics tool alone.
Conclusion
It’s not a question of whether you need a data warehouse or even whether the ROI is there. It’s more about the specific tools and tech you’re going to implement from the get-go – switching a vendor later is a lot of effort and costly, too.
Our recommendation is to avoid CDPs and other fancy buzzword tools and instead get something more robust – BigQuery as your data warehouse, a set of data pipelines, a BI tool for reporting and then go from there.
Data engineers at Reflective Data would be more than happy to answer your questions in the comments below or hop on a quick free consultation session.